Advanced Data Analytics
Course
Date
Number of words
Name
Institution
Advanced-Data Analytics
Advanced-Data Analytics代写 Terminology:1.Trend analysis 2.Behavioral analytics 3.Contextual data 4.Data visualization 5.Risk analysis 6.Financial volatility
Terminologies Advanced-Data Analytics代写
1. Trend analysis
Trend analysis uses past data to make predictions on the market behaviors in the future¹. Every data analytics specialists need to have knowledge of the analysis data trends in the market and make a rational financial decision. Therefore, it is crucial to be well-oriented with how and why trend analysis in identifying and predicting market trends.Advanced-Data Analytics代写**格式
Generally, trend analysis is a comparative analysis of data over some time to predict future behavior. The primary trend analysis in the stock market is to forecast the movements of stock prices. A stock analyst uses past information and data to give an idea of what to expect from stocks in the future. As such, a consultant can use trend analysis to advice a client when to enter the market and when to exit. The analysis can also help the trader spot potential problems in stock before they occur.
2.Behavioral analytics Advanced-Data Analytics代写
Involve an analysis of people’s actions in the buying and selling of stocks. Consumer behavior helps in the prediction and optimization of stock trading². The presence of big data has facilitated the analysis of people behavior and hence, likely to provide an accurate prediction on market performance and other issues. A data analyst takes a keen interest in what people say and do regarding the stock market because their actions and communications have an impact on the stock exchange decisions.Advanced-Data Analytics代写**格式
3.Contextual data
This is the data that provide stock market context. The data is used to give a broader understanding of the specific stock and placing it in a larger picture. Contextual data gives the historical performance of certain stock to facilitate future decision.
4.Data visualization Advanced-Data Analytics代写
It involves the use of graphical representation to present information and data about stock market performance. Visualization is used to make it easy for the user to recognize patterns and exceptions and hence make quick interpretations.
5.Risk analysis
It is the process of identifying and quantifying issues with the stock market and making estimations about their impact. Risk assessment in the stock market is a critical function of a data analyst to a client for there to be an informed judgment.
6.Financial volatility Advanced-Data Analytics代写
It is the statistical measure of the dispersion of returns of a specific stock in the market. A data analyst tries to measure whether the stock volatility is high or low and advice the client accordingly. High volatility is associated with high-risk stocks.
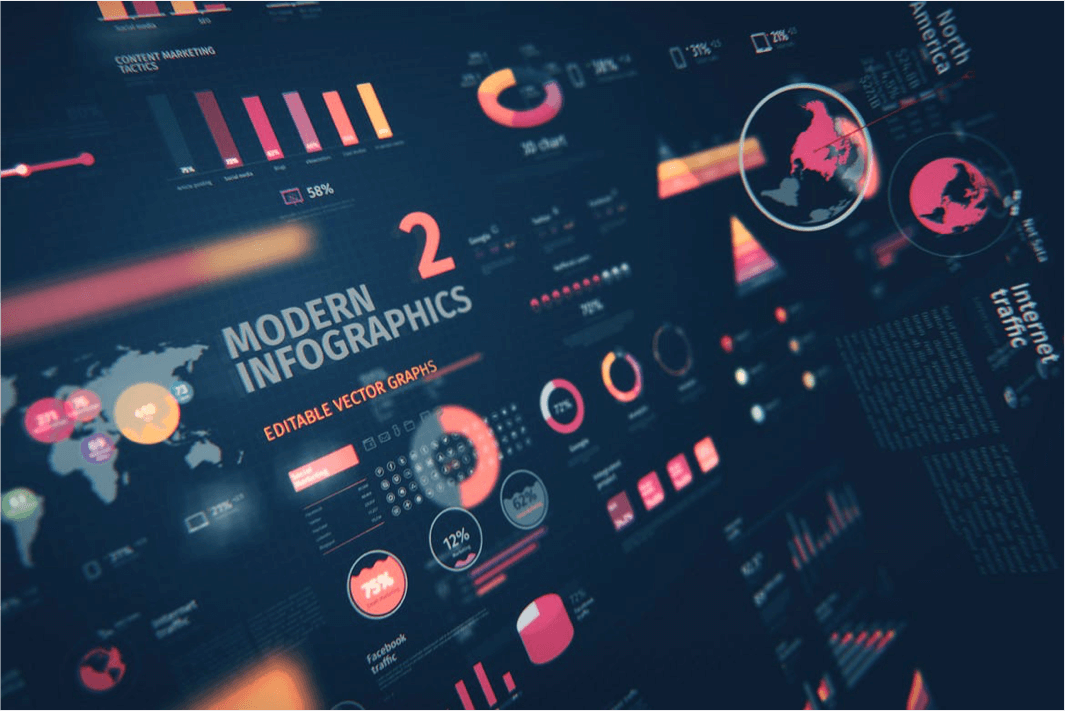
Bibliography
¹J.Patel et al., “Predicting stock and stock price index movement using trend deterministic data preparation and machine learning techniques.” Expert Systems with Applications vol. 42, no. 1, 2015, p. 259-268.
²G.Nabieu, “Model Behavior Analysis of Stock Market Indicators and Listed Companies: Evidence from the Ghana Stock Exchange: Automated versus Floor Trading.” International Journal of Business and Managementvol. 9, no. 11, 2014, p.234.
Critical Evaluation of Common Data Analytics Methods Advanced-Data Analytics代写
1.Mean
It is the average where the observations are divided by the number of observations made, as shown in the formula below. Although it is an essential tool in data analysis, it is not sufficient to facilitate rational decision. Mean is used together with mode and median to give more information about the data set. Also, mean does not provide an accurate answer for nuanced decision in extensive data which is likely to be skewed.Advanced-Data Analytics代写**格式
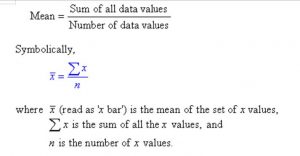
2.Content analysis Advanced-Data Analytics代写
The method is used to measure the overall theme(s) in qualitative data. The analysis helps parse textual data such as questionnaires, interviews, and open-ended surveys to find the most common thread. The method is most appropriate in quantitative data but not qualitative data. Therefore, it cannot provide numerical measures that assist in the decision. The method mostly biased as it is based on user opinion and feelings which might not represent the population.
3.Regression analysis
The method is used to measure the statistical relationship between two or more variables. It is used to measure a trend that might exist in a set of data, as shown below. But, the method is not very nuanced since it may ignore essential outliers.Advanced-Data Analytics代写**格式
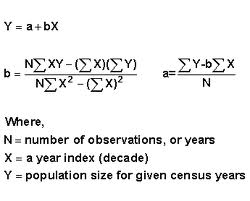
4.Monte Carlo Simulation Advanced-Data Analytics代写
The technique is used to make predictions on the effect of unpredictable variables on a specific factor by use of the probability model and hence help in making predictions for risks and uncertainty. It is used to simulate random data to get several possible outcomes. Monte Carlo Simulation is critical for testing data uncertainty in results.
5.Hypothesis testing
The method is used to test whether a particular premise is true for data set or population. It is also considered during data analysis and statistics to test the statistical significance of the results. However, the method is faced with various errors, including placebo and Hawthorne effects. Nevertheless, the technique is essential in data analysis and decision making.
Importance of data analytics Advanced-Data Analytics代写
i. Improve efficiency
The data collected and analyzed Calcutta Analytic reflects both internal and external state of the business. Therefore, the interpretation of the data will help to measure the performance of employees and the business as a whole.
ii. Market understanding Advanced-Data Analytics代写
Calcutta Analytic uses an improved algorithm, which has enabled collection and analysis of extensive data. The use of data from a variety of sources and people facilitate in formulating a marketing strategy.
iii. Cost reduction
Big data technologies like cloud analytics and Hadoop used by Calcutta Analytic have the advantage of low cost, especially when broad data is involved.Advanced-Data Analytics代写**格式
iv. Better decision-making
The consultant uses high-speed in-memory analytics and Hadoop as well as a variety of data sources which enable instant data analysis. The process saves time, and hence, decisions are made more efficiently and quickly.
v. Understand the industry Advanced-Data Analytics代写
The data collected, analyzed, and presented is useful in the understanding of the industry. Thus, the business can decide for future operations.
Guidebook
Evaluation of Analytical Models Data Preparation Processes Advanced-Data Analytics代写
The use and creation of analytical models have become a common practice in data analysis³. Analytical models are used to get a solution to the statistical problem using mathematical calculations. That is, the results of the model are used to describe a certain phenomenon in an organization that can be expressed as a mathematical analytic function⁴. Analytical models have continued to evolve in a deeper understanding of mathematical use and application of results.Advanced-Data Analytics代写**格式
The methods of analytical modeling are not definite since data scientists are not reducible to articulate rules. In the presence of extensive data, analytical models are essential in simplifying the method for arriving at the research solution. In the advent of big data, the data scientists are supposed to come up with models that will enable them to analyze and interpret data sets. One of the commonly used analytical data modelings is Cross Industrial Standard Process for Data Mining (CRISP-DM)⁵.Advanced-Data Analytics代写*格式
On the other hand, the data preparation process is all tasks that involve acquisition, processing, and cleansing data⁶. Data preparation include “accessing, loading, structuring, purging, unifying (joining), adjusting data types, checking fields to verify values and ensure uniformity in data.” The data is used in the predictive analysis model, which help to improve business effectiveness and success. The model uses a combination of data, statistics, and machine learning techniques. As such, the following are steps for building a successful predictive analysis model.Advanced-Data Analytics代写**格式
Bibliography
³R.Grossman and K. Siegel. “Organizational models for big data and analytics.” Journal of Organization Design vol. 3, no. 1, 2014, p. 20-25.
⁴W.Lin et al., “Combining predictive models in predictive analytical modeling.” U.S. Patent 8,370,280, issued February 5, 2013, p. 5.
⁵U.Shafique and Q. Haseeb, “A comparative study of data mining process models (KDD, CRISP-DM and SEMMA).” International Journal of Innovation and Scientific Research vol. 12, no. 1, 2014, p. 217-222.
⁶S.Kwak and Jong Hae K, “Statistical data preparation: management of missing values and outliers.” Korean Journal of anesthesiologyvol. 70, no. 4, 2017, p. 407.
a. Understand the Objective Advanced-Data Analytics代写
A good predictive model is built on a clear understanding of the organization’s objectives as well as the data analytics objectives⁷. It is crucial to note various purposes that organizations have including management of risks and frauds, financial prediction and modeling, social media influence, marketing strategy, operational efficiency, and among others. A data analyst is required to choose the objective accordingly. As such, the goals of data analysis are based on organizational objectives.Advanced-Data Analytics代写**格式
⁷D.Groebner et al., Business statistics. Pearson Education UK, 2013, p.50.
b. Identify the Problem
The primary aim of the model is to identify the organizational problem. Thus, a data analyst identifies the problem in the organization that the data analysis seeks to solve. The outcome of the data analysis is vital since it helps workers and managers to approach the issue in the organization.
c. Determine the Process Advanced-Data Analytics代写
Data analysis follows a predetermined process. The process can, therefore, be analyzed to identify improvement opportunities. A data analyst should assess the various methods available choose one and make changes to implement the results of the model.
d. Identify Performance Metrics
Key performance indicators are essential to orient the organizational operations and management to the targets⁸. A data scientist should identify right performance metrics that can quantitatively measure organizational goals and give room for improvements in the system. The parameter should also allow adjustments or adoption of a different approach in case the action is taken fails to yield expected outcomes. Advanced-Data Analytics代写**格式
⁸D.Parmenter, Key performance indicators: developing, implementing, and using winning KPIs. John Wiley & Sons, 2015, p. 10.
e. Select and Prepare Data for Modeling Advanced-Data Analytics代写
A data scientist needs to select data that are appropriate and align with a business organization as well as target modeling. Thus, data selection requires a proper understanding of the business objective and model to be used. A data analyst has three types of data to choose for modeling, including psychographic, demographic, and behavioral variables. The critical part in data preparation is analysis format to be used. As such, the model is trained using past data to ensure the suitable format is used, and data clean-up is done correctly. As a result, variables become well-defined, and multiple data sets merged.Advanced-Data Analytics代写**格式
f. Model Development Methodology
Model Development Methodology “is used to structure, plan, and control the process of developing a system in an organization.” As a business consultant, there are various development methodologies to adopt in data analytics such as “agile software development, dynamic systems development model, feature-driven development, rapid application development, systems development life cycle, etc.” The various approaches available are for minimizing risks in modeling by the use of software in short iterations where the data analysts can evaluate results.
g. Random Data Sampling Advanced-Data Analytics代写
Data sampling is used to make selections, manipulations, and analysis of data subsets to identify patterns and trends⁹. Traditionally, data are split into training and test sets. Extensive data set is used to create the model while the remaining ones are used as test sets of the results from the model. The process is used to create an executable model of outcomes in a manner that is efficient and effective.Advanced-Data Analytics代写**格式
⁹L.Palinkas et al., “Purposeful sampling for qualitative data collection and analysis in mixed method implementation research.” Administration and policy in mental health and mental health services research vol. 42, no. 5, 2015, p. 533-544.
h. Data Management Program
Data management is critical in ensuring the success of the data preparation process. A data analyst requires to implements a program for data governance, which assists in assuring data analytics quality and consistency. A good data management program forms a foundation for data management. Also, organizational management contributes to an effective and efficient model performance.
i. Implementation of the Model Advanced-Data Analytics代写
The model is implemented in the data analytics system after it has been developed and validated. Model implementation in the system has several methods, including “account management systems, decision-making systems, customer relationship management systems, analytics platforms, collection systems, etc.”
j. Building and Deployment of the Model
A robust data analytics model is run on many algorithms. Several algorithms give various possible outcomes from which the most efficient result is selected. After development, the model needs to be deployed in data analytics and decision-making. There are three methods of implementing a data analytics model, including model scoring, integration in reporting, and integration in business operations¹⁰.Advanced-Data Analytics代写**格式
¹⁰S.Sagiroglu and D. Sinanc, “Big data: A review.” In 2013 International Conference on Collaboration Technologies and Systems (CTS), IEEE, 2013, p. 42-47.
Issues in Data Presentation Advanced-Data Analytics代写
Businesses want to create value by adopting data modeling and curation practices. However, to achieve efficiency in data analytics, data analysts should consider several challenges for making the modeling process work effectively. The most significant problem is creating a correlation of data with real-world and integrates it with business processes and analytics software. Other issues include data curation and modeling across disparate sources and data stores.
i. Ensuring data quality
Ensuring data quality is a challenge mainly owing to extensive data that require additional effort¹¹. Traditionally, the data quality assurance involves hand-coded business logic, which is challenging to maintain and scale. Automated data cleaning and preparation is an investment in data engineering that is expensive. The process can be impeded if the managers fail a better understanding of the project value.Advanced-Data Analytics代写**格式
¹¹N.Anney, “Ensuring the quality of the findings of qualitative research: Looking at trustworthiness criteria.” Journal of Emerging Trends in Educational Research and Policy Studies (JETERAPS) vol. 5, no. 2, 2014, p. 272-281.
ii. Identifying contributors to dirty data
Data accuracy needs to be assured onset at the source. If the modeling is based on inaccurate data, it will give wrong results and decisions and will crumbles¹². Data inaccuracy occurs at the time of collection and acquisition or during cleaning. Therefore, if the business decision is made based on wrong data, it could result in severe repercussions to the business.Advanced-Data Analytics代写**格式
¹²J.Wang et al., “A sample-and-clean framework for fast and accurate query processing on dirty data.” In Proceedings of the 2014 ACM SIGMOD international conference on Management of data, ACM, 2014, p. 469-480.
iii. Enabling fitness for purpose
The data prepared for analysis need to be correct and trustworthy for the intended use and according to business objectives¹³. Accurate and dependable data is challenging to have and require contextual effort. A data analyst should work to ensure that the data used does not have a lot of outliers, which will reduce fitness.Advanced-Data Analytics代写**格式
¹³M.Hodkiewicz and N. Montgomery. “Data fitness for purpose: assessing the quality of industrial data for use in mathematical models.” In 8th International Conference on Modelling in Industrial Maintenance and Reliability, Institute of Mathematics and its Applications, Oxford, 2014, p. 125-130.
iv. Breaking down data silos
Data break down is another challenge which is based on the legacy architectures and hence undermines the effectiveness of predictive analytic models¹⁴. Also, other data sources like premium data source and machine learning have gained popularity over the last few years. Another challenge is created by data privacy. Therefore, data analysts should know the data available to them and master the data-driven culture.Advanced-Data Analytics代写**格式
¹⁴J.Wilder Edd., “Breaking down data silos.” Harvard Business Review, 2016, p.3.
v. Avoiding Las Vegas effect
Data users have choices of platforms to use to create new silos like using SaaS-specific analytics and model data sets outside that of centralized data engineering efforts. There is a need for balance between ad hoc analytics platforms used so that there are centralized data management practices. For instance, the use of Tableau to fix errors in data may fix the problem but create a new data silo. It is estimated that data analysts use only 19 percent of the time to analyze data and 81 percent in data acquisition, preparation, and protection.
vi. Starting fresh or cleaning old data Advanced-Data Analytics代写
Organizations are faced with sunken cost bias when deciding on a curation and data modeling process. Businesses have enormous data that they do not have perfect knowledge of how the data fit their overall strategy and objective. It might be difficult for managers when a data analyst proposes throwing away the data for cost reduction instead of cleaning them. Therefore, data analysts are faced with the challenge of the trade-off between taking in more data and cleaning the already existing data.
vii. Understanding what the business cares about
Failure to understand the value of data analysis to business lowers the motivation for data curation. A data might be wrong for a particular purpose but useful for another application. For instance, bad sales data that has inaccurate customer details is bad for sales, but the same data might be relevant in implementing artificial intelligence to identify sales challenges.
Visualization Methods Advanced-Data Analytics代写
Data visualization is an essential approach in the applied analytic model and involves building visual representations of information¹⁵. Visualization involves the communication of data information clearly and efficiently to the intended audience. Data visualization can take the form of lines, bars, or dots that have quantitative meaning. Therefore, a data analyst is required to choose the right method to make data understandable and valuable.
There are various reasons why data visualization is extremely effective and useful in analytics models. It is difficult for humans to understand and interpret digits in a large data set. Extensive data set needs to be presented in meaningful and straightforward forms that are understandable to the intended audience. Data visualization comes into play when converting data into pictorial representations. Another reason for data visualization is that humans are limited in the comprehension of factual data in terms of numbers. Therefore, raw data is difficult to understand unless converted to more appealing forms like graphs.Advanced-Data Analytics代写**格式
¹⁵A.Telea, Data visualization: principles and practice. AK Peters/CRC Press, 2014, p. 30.
Methods of Visualization Advanced-Data Analytics代写
i. Charts:
They are the most commonly used method of data visualization. Charts include a pie chart, line chart, and bar chart. It is easy to develop charts for one or several data sets. However, some people find it challenging to understand pie chart while comparing to the line and bar chart. The line chart is simple and more appealing. It is used to show the relationship between two variables. Combo chart and bar chart are used to compare two different patterns
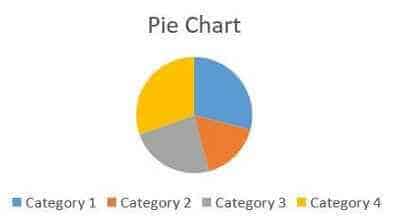
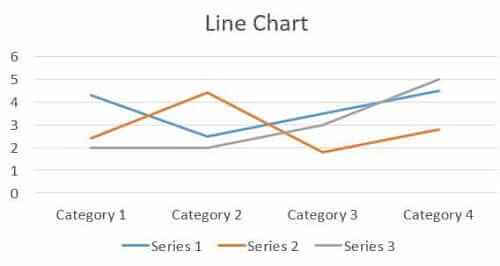
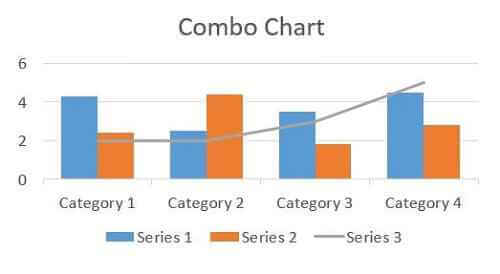
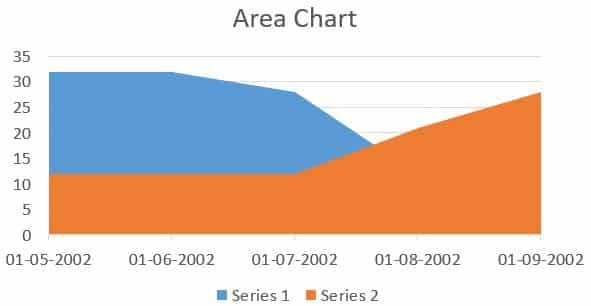
ii. Maps:
The visualization allows the positioning of variables on a specific position on the map or areas such as geographical maps, building plans, website, etc. The commonly used maps visualizations are heat maps and dot distribution.
Heat maps are used in various forms of analytics of websites, software makers, research, etc. It shows how activities/entities are concentrated in a particular area.
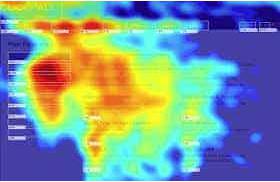
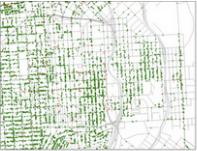
iii. Diagrams and matrices:
Diagrams are used to show the relationship and links between complex data. The links and relationships between data can be hierarchical, multidimensional, and tree-like. On the other hand, the matrix uses to visualize big data and shows correlations between multiple constantly updating data sets.
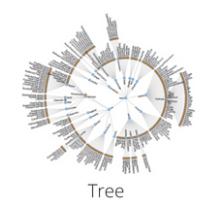
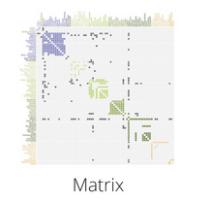
iv. Network diagram for unstructured data:
It represents the relationships as nodes and ties. The network shows how components should connect. The method is used to analyze social networks or mapping product sales across geographical areas.
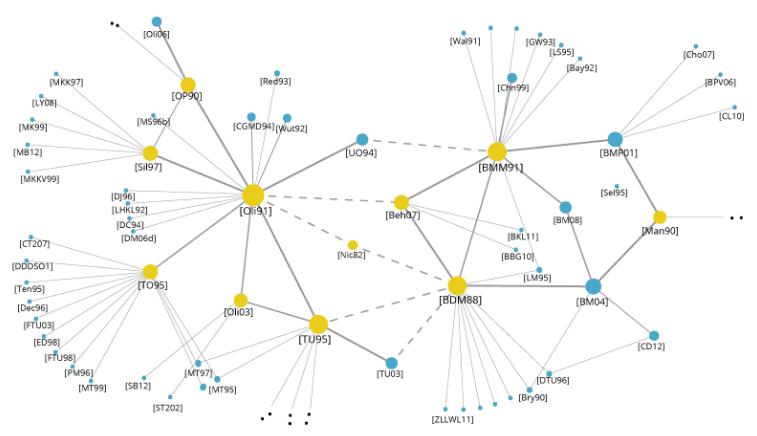
v. Scattered 3 D plot:
The visualization represents the distribution of elements in 3D nature. The visualization helps to see data in three dimensions and hence gives better insight into the relationships between various variables.
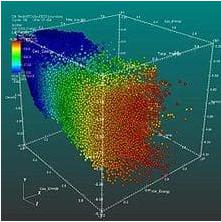
Bibliography
1-7
- Grossman, R. and K. Siegel. “Organizational models for big data and analytics.” Journal of Organization Design 3, no. 1, 2014, p. 20-25.
- Lin, et al., “Combining predictive models in predictive analytical modeling.” U.S. Patent 8,370,280, issued February 5, 2013, p. 5.
- Shafique, U. and H. Qaiser, “A comparative study of data mining process models (KDD, CRISP-DM, and SEMMA).” International Journal of Innovation and Scientific Research 12, no. 1, 2014, p. 217-222.
- Kwak, Sang Kyu, and Jong Hae Kim. “Statistical data preparation: management of missing values and outliers.” Korean Journal of anesthesiology 70, no. 4, 2017, p. 407.
- Groebner, D. et al., Business statistics. Pearson Education UK, 2013, p. 50
- Parmenter, David. Key performance indicators: developing, implementing, and using winning KPIs.John Wiley & Sons, 2015, p. 10.
- Richey, C. and D. Klein, Design and development research: Methods, strategies, and issues.Routledge, 2014, p. 120.
- Palinkas, L. et al., “Purposeful sampling for qualitative data collection and analysis in mixed method implementation research.” Administration and policy in mental health and mental health services research 42, no. 5, 2015, p. 533-544.
8-14
- Sagiroglu, S. and D. Sinan, “Big data: A review.” In 2013 International Conference on Collaboration Technologies and Systems (CTS), IEEE, 2013, pp. 42-47.
- Anney, N, “Ensuring the quality of the findings of qualitative research: Looking at trustworthiness criteria.” Journal of Emerging Trends in Educational Research and Policy Studies (JETERAPS) 5, no. 2, 2014 p. 272-281.
- Wang, J. et al., “A sample-and-clean framework for fast and accurate query processing on dirty data.” In Proceedings of the 2014 ACM SIGMOD international conference on Management of Data, ACM,2014, p. 469-480.
- Hodkiewicz, M. and N. Montgomery, “Data fitness for purpose: assessing the quality of industrial data for use in mathematical models.” In 8th International Conference on Modelling in Industrial Maintenance and Reliability, Institute of Mathematics and its Applications, Oxford, 2014, p. 125-130.
- Wilder-J, Edd. “Breaking down data silos.” Harvard Business Review,2016, p. 3.
- Telea, , Data visualization:principles and practice. AK Peters/CRC Press, 2014, p. 30
更多其他:数据分析代写 润色修改 代写案例 Case study代写 心理学论文代写 毕业论文代写 论文代写 Essay代写 研究论文代写 Academic代写 Review代写 网课代修 代写CS
您必须登录才能发表评论。