AI in Healthcare
AI in Healthcare代写 This paper investigates Artificial Intelligence techniques applied in healthcare. Our project examines in detail four case studies.
Abstract
Objective:
This paper investigates Artificial Intelligence techniques applied in healthcare. Our project examines in detail four case studies, outcome and limitations, methodology, compare and contract and most importantly recommendations to solve complex clinical problems within different industries.
Background:
Artificial intelligence (AI), study the process of enabling machines to skillfully perform intelligent tasks, without explicitly being programmed for those tasks. Recently, AI systems have neared or surpassed human performance in several tasks in different areas. Health care is one of the areas where Artificial intelligence has demonstrated exceptional results. Artificial intelligence has brought a shift to healthcare, powered by increasing availability of healthcare data and rapid progress of analytics techniques.AI in Healthcare代写**成品
The primary goal of health related AI applications is to analyze relationships between prevention or treatment techniques and patient outcomes. AI programs have been developed and applied to situations such as diagnosis, treatment protocol development, drug development, personalized medicine, and patient monitoring and care.
Methods:
Discussion:
Artificial intelligence (AI) is an attempt to simulate the intelligent behavior in computers with intelligent human behavior. The goal of (AI) in healthcare is to allow faster, accurate access to a lot of data. The hope is that this will reduce costs and improve clinical outcomes. AI in healthcare will assist providers with drug discovery, recommendations and treatments. With AI being newly introduced in some areas, more research is needed to determine the effectiveness of AI in various healthcare settings.
Introduction AI in Healthcare代写
The incorporation of Artificial intelligence into different aspects of our day to day lives ranging from transportation to the health industry from the late twentieth century has been rapid and promising in its potential to improve human life.
In the healthcare industry, AI-powered applications and technologies can be used to the quality of life of patients and people around the world and additionally. Improve the health results (“Artificial Intelligence and Life In 2030”, 2016). Some technology companies and research studies have already tapped into this market and have come with various applications and technologies whose primary aim is to improve health diagnostic methods. And one such company is IBM with its revolutionary technology known as the IBM Watson.AI in Healthcare代写**成品
Watson for Drug Discovery reveals connections and relationships among genes, drugs, diseases. And other entities by analyzing multiple sets of life sciences knowledge, as a way to help researchers uncover potential new therapies and find new uses for existing medicines (“What is Watson,” 2018). Watson has played a critical role in helping academic institutions, biotech companies and pharmaceuticals to repurpose drugs or identify new drug targets. Innovations like this are set to take healthcare to the next level through the democratization of the access to personal health data and innovative ways of bringing computer science and biomedicine together.
The Berg Interrogative Biology Informatics Suite is an automated data-processing instrument for generation of actionable hypotheses using data generated exclusively via the Berg Interrogative Biology approach. AI in Healthcare代写
The Berg Interrogative Biology is a platform that systematically interrogates the biological environment in proprietary in-vitro and in-vivo biological model systems. The platform offers a new direction and impetus to drug discovery initiatives by primarily engaging the juxtaposition of the disease biology and normal cellular (homeostatic) biology, which is comprised of characteristic signatures including genes, proteins, lipids, metabolites and their network of expression. The disease modeling efforts involve extensive ex vivo experimental work with human material such as cell extracts, tissue samples, and cell lines (Niven R Narain and Anne R Diers, 2016).AI in Healthcare代写**成品
BERG invented cellular interrogation mechanisms by environmental, chemical and biochemical perturbants that induce disease-specific molecular signaling.
Such signaling mechanisms can be deciphered by BERG artificial intelligence engine, bAIcis. Over the years, BERG developed an extensive therapeutics and diagnostics pipeline of assets by applying Interrogative Biology in disease areas such as cancer, diabetes, neurology, and autoimmune disease. In parallel, BERG has validated this method through rigorous and manual experimental work in its vitro laboratory (BERG Analytics, 2017).
Novel biomarkers that are currently in development for PrCa include the TMPRSS2-ERG fusion. AI in Healthcare代写
PCA3 and AMACR, each of which have been described for use alone or use in combination to improve the diagnosis of PrCa. However, a major disadvantage of these biomarkers is the need for core biopsy specimens for assessment. Thus, while these tests alone or in combination with PSA are clinically useful, discovery and development of biomarkers that can be detected in biofluids or any other easily available matrix would provide an advantage over existing markers in development.AI in Healthcare代写**成品
Proteomic profiling of the secretome of cancer cells is a promising strategy that is employed to identify novel biomarkers for various cancers including PrCa.
The ‘secretome’ is a term that was first presented by Tjalsma et al. based upon their findings from genome-wide screening of putative proteins that are secreted by Bacillus subtilis. As secreted proteins may theoretically enter bodily fluids, their potential ability to be detected using noninvasive methods makes them a highly attractive target for biomarker discovery. Therefore, in the present report a novel data-driven systems biology-based approach was used to identify potential biomarkers for PrCa from interrogation of the secretome of PrCa cells.
Artificial intelligence techniques have the potential to be applied in almost every field of medicine. AI in Healthcare代写
It will respectively be that engine that drives improvements across the care continuum. For instance, IBM Watson for Oncology (WFO) is a cognitive computing system that provide evidence-based treatment options for cancer. This system uses biomarkers in breast cancer which are essential contributors to therapeutic assessment. In addition to standard prognostic factors, three established predictive biomarkers, estrogen (ER) and progesterone (PR) receptors and human epidermal growth factor receptor 2 (HER2) status, are utilized for treatment decisions. Calculation of individual response predictions remains poor without inclusion of the gene expression dataset (Prognostic and predictive, 2010).AI in Healthcare代写**成品
In addition with that, The EndoPredict (EP) assay is an RT-qPCR-based 12 gene test based on RNA from FFPE tissue in hormone receptor-positive, HER2-negative, nodal-negative and-positive breast cancer managed that has been designed to integrate genomic and clinical information (Clin Cancer Res,2011). EP scores with clinicopathologic risk factors results in an EPclin score that facilitates the identification of risk groups. When a question is programed into the system, WFO treatment options are presented in three categories: ‘Recommended’, ‘For consideration’, and ‘Not Recommended’. This system can be compared to a car navigation system, meaning that it helps clinicians reach therapeutic goals rapidly and safely.
Literature Review AI in Healthcare代写
Bakkar et al. (2017) used IBM Watson to go through all the RNA-binding proteins (RBPs) and detect additional RBPs altered in amyotrophic lateral sclerosis (ALS). ALS is one of the most devastating neurodegenerative diseases with no known cure or treatment. So far, research has revealed numerous RBPs altered In ALS with 6 RBPs showing abnormal expression in ALS without any mutations and 11 RBPs with mutations causing familial forms of the condition. This study was to find new RBPs that had no known link to ALS since the human genome contains 1542 RBPs. Therefore, there was a high possibility of discovering additional RBPs altered in ALS (Bakkar et al., 2017). IBM Watson was successful in identifying these additional RBPs and giving hope to the use of artificial intelligence in the discovery of other complex neurological disorders and eventually their treatments.
Buus et al. (2015) utilize the system to study 95 patients that were treated for early breast cancer at Gachon University Medical Center in South Korea between Dec 2015 and July 2017.
It was approved by the Institutional Review Board and involved no more than minimal risk for the subjects. The study group was limited to HER2-negative and estrogen receptor positive breast cancer. All patients went through breast conserving surgery or total mastectomy and also a lymph node biopsy. Among the 95 patients and tumor characteristics, 83 (87.4%) were diagnosed with node-negative disease and 12 (12.6%) with node-positive disease. This study was conducted to examine 95 patients using WFO, and it outputs compared to real clinical practice. It focused on the hypothesis that WFO alone cannot effectively determine whether or not chemotherapy is essential for the subset of ER-positive, HER2-negative BC patients.AI in Healthcare代写**成品
This study reports the identification of a panel of potential biomarkers using the Berg Interrogative Biology platform, a novel non-hypothesis driven approach to characterize disease specific molecular signatures for the identification of physiological drivers of disease etiology. Filamin B (FLNB) and lymphocyte antigen 9 (LY9) were two of several markers identified from a high confidence causal network of prostate cancer model using the Berg Interrogative Biology platform. Both FLNB and LY9 were validated in a small case-control retrospective study in human serum samples of patients with prostate cancer and age-matched normal controls.
Residual lithium-heparin plasma was collected from patients with suspected PrCa. AI in Healthcare代写
The criteria for inclusion were: elevated PSA results (≥2.6 ng/ml), age 45–70 years and ≥700 µl of sample. Samples were excluded if patients had any cancer diagnosis prior to collection. Biopsy information was accessed 6–8 weeks after sample collection. The diagnosis of benign, prostatic intraepithelial neoplasia or adenocarcinoma was noted. A Gleason score was recorded for patients with prostate adenocarcinoma.
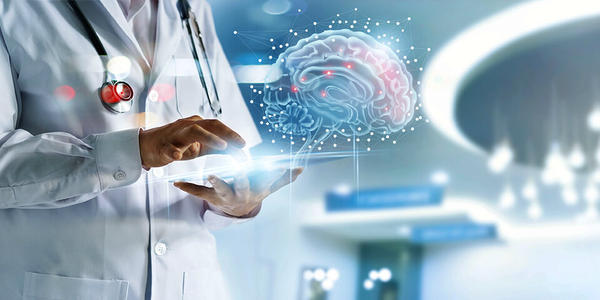
Case Study 1: IBM Watson platform for Drug Discovery AI in Healthcare代写
Bakkar et al. (2017) obtained tissue samples from the Barrow Neurological Institute ALS Tissue Bank, the University of Pittsburgh ALS Tissue Bank and the Target ALS Human Postmortem Tissue Core. Collection of tissue samples was after evidence of the provision and approval of informed consent by the Dignity Health Institutional Review Board and the University of Pittsburgh Institutional Review Board (Bakkar et al., 2017). Laser capture microscopy captured at least 120 Purkinje cells per slide in one hour to minimize RNA degradation. RNAqueous micro total RNA isolation kit from Ambion (AM1931) extracted RNA while Superscript VILO synthesized cDNA. After this, analysis of the extracted RNA used real-time RT-PCR.AI in Healthcare代写**成品
Quality and Safety
The IBM Watson measures the quality of data according to the pre-set data sets configured before the analysis. Therefore, it is able to read and assess the data for quality before it providing the results. the IBM Watson is a high-quality technology that is revolutionizing the healthcare system. For safety, the configuration of multiple layers of data on the IBM Watson Analytics ensures that one’s data is safe and additionally. Measures are in place to ensure that data on Watson cannot be shared without proper permission.
Data Security and Privacy AI in Healthcare代写
When using the Watson services on the IBM Cloud, the ability to combine datasets with other licensed, IBM-owned, or public data sets to gain more valuable insight is possible. However, strict safeguards are in place to guard any data breaches on the IBM Watson with the use of data encryption methods and proprietary consent management modules.
Usability and Acceptance
The IBM Watson before being used for its intended purpose in this study was put through a series of tests to determine its proficiency and validity. The tests like the leave-one-out (LOO) cross-validation revealed that the predictive power of this technology was strong. Therefore, it was capable of detecting the RBPs (Bakkar et al., 2017). The IBM Watson has been fairly accepted in the scientific world because of the promise it holds for scientists and researchers.
Outcome and Limitations AI in Healthcare代写
The IBM Watson was able to identify five proteins that had no known previously linked IN altered ALS. However, the limitations of IBM Watson are the generation of a false positive. IBM Watson depends on gene annotations of the published literature for its text-based analysis. In the study, Watson ranked hnRNPH2 number 6, exhibited few changes in ALS. But was found to have a similar annotation nomenclature within the published literature as hnRNPH/hnRNPH1, which had links to ALS. The instance of common annotations most probably led Watson to infer that hnRNPH2 was equivalent to hnRNPH and hnRNPH1. That led to the generation of a false positive in our analysis.AI in Healthcare代写**成品
While a rigorous disambiguation of gene annotations for the study continued work on gene annotations. It will aid future gene-based studies using IBM Watson (Bakkar et al., 2017). Additionally, IBM Watson’s base on semantic likeness to a known set of proteins. For example, Watson ranked DDX58, a protein already linked to ALS as number 769 making it a false negative.
Case Study #2 Watson for Oncology AI in Healthcare代写
In the study by (Liu et al., 2018), Watson for Oncology (WFO) recommendations for lung cancer patients were compared to recommendations of expert oncologists in China. Research was done using a retrospective study. There were a 182 patient records assessed to determine if using the artificial intelligence, WFO, was beneficial and how to make WFO more suitable for patients in China. 33 of the cases were not supported by WFO. Inpatients in the center, lung cancer patients, those who received antitumor treatment for the first time and admissions between September 2017 and December 2017 were included in the study (Liu et al., 2018). The setting was Second Xiangya Hospital in China. The hospital services more that 30,000 cancer patients every year.
Quality and Safety
Measures were used to determine what percentage of recommendations made by WFO were consistent with the multidisciplinary team. When the WFO recommendations were not consistent with the team recommendation, patients were administered two China-specific treatments. Some question to what extent WFO is suitable for cancer patients. Also, what is the specific value of WFO. There are several steps taken to ensure the accuracy of the information. After the information and treatments were collected, doctors manually input the information into WFO and recorded the recommendations. Next two doctors compared the results from WFO and the treatment that was given. Then a team of specialists converge to discuss treatments given. AI in Healthcare代写**成品
Data Security and Privacy AI in Healthcare代写
Lie et al. (2018) defines WFO as a clinical decision-support system for oncology therapy selection. “WFO has stored and indexed literature, protocols, and patient charts; has learned from test cases and experts from Memorial Sloan Kettering Cancer Center (MSKCC). And can apply computational reasoning approaches to specific cases” (Liu et al., 2018, p. 3). Top oncologists at MSKCC verify all information and the cutting edge information is updated every 1-2 months.
All cases are not supported by the WFO. In those cases, when information is input, it is not processed and there are no recommendations. Supported case recommendations include recommended, for consideration and not recommended. Recommended means that there is strong evidence suggesting the treatment. For consideration means that the treatment is potentially suitable for the patient. Not recommended means there are no recommended treatments, however, WFO will give the most standard treatment for the specific case.AI in Healthcare代写**成品
Usability and Acceptance
There were mixed results during the study. Although over 80% of the patients’ cases were supported by WFO, 18.1% of the patient cases were not supported. The numbers also varied amongst different types of lung cancers. Researches suspect the reason for unsupported cases were due to complicated cases. And the inability of current technology of WFO to support the cases (Liu et al., 2018).
While the recommendations were not as high as expected, consistent recommendations came with evidence based treatments. This information was useful to oncologists allowing them to determine whether the treatment would be given. WFO also provides oncologists with survival rates, incidence of adverse effects and other information related to selected treatment. “WFO can markedly shorten the time that junior doctors must spend consulting relevant literature. Thus improving their ability to make accurate diagnoses and treatment recommendations in a short time” (Liu et al., 2018, p. 6).
Outcomes and Limitations AI in Healthcare代写
The study had multiple limitations because it was a retrospective observational study with no control group (Liu et al., 2018). The included patient records were from patients at different stages of cancer. This could have affected the outcome pertaining to the success and failure of WFO. Also, there were more men included in the study which could have altered the recommendations. AI in Healthcare代写**成品
All patients underwent breast conserving surgery or total mastectomy and also a lymph node biopsy. Formalin-fixed paraffin-embedded (FFPE) tissue samples were derived from patients with primary ER-positive and HER2-negative breast cancer. All FFPE tumor blocks were collected at the time of surgery prior to therapy. Total RNA was extracted from FFPE tissue sections using a silica-based, fully automated isolation method. All samples were subjected to quantitative one-step SuperScript III PLATINUM reverse transcriptase PCR with ROX.
The approach used in this study combined in vitro perturbation biology and proteomic analysis with in silico Bayesian causal analysis and delineation of subnetworks.
The metastatic prostate cancer cell line, PC-3, was exposed to perturbations mimicking the tumor microenvironment (poor oxygenation, low pH and diminished nutrient state) and conditioned media were subjected to proteomic analysis 24 and 48 h after culture. Proteomic data were analyzed using a Bayesian network inference approach, in which each particular factorization and the choice of parameters are a distinct probabilistic model of the process that created the observed experimental data. Another approach used was to determine that Subnetworks linked to the functional variables were ranked based on their Burt’s constraint score metric. Which measures the extent to which nodes (proteins) are connecting to unconnected modules and the relationship redundancy within each of these modules.
Case Study #3 BERG Interrogative Biology AI in Healthcare代写
The approach used in this study combined in vitro perturbation biology and proteomic analysis with in silico Bayesian causal analysis and delineation of subnetworks. The metastatic prostate cancer cell line, PC-3, was exposed to perturbations mimicking the tumor microenvironment (poor oxygenation, low pH and diminished nutrient state) and conditioned media were subjected to proteomic analysis 24 and 48 h after culture. Proteomic data were analyzed using a Bayesian network inference approach, in which each particular factorization and the choice of parameters are a distinct probabilistic model of the process that created the observed experimental data.AI in Healthcare代写**成品
Another aproache used was to determine that Subnetworks linked to the functional variables were ranked based on their Burt’s constraint score metric. Which measures the extent to which nodes (proteins) are connecting to unconnected modules and the relationship redundancy within each of these modules.
Quality and Safety
All procedures were performed in accordance with the ethical standards of the responsible committee on human experimentation (institutional and national). And with the Helsinki Declaration of 1975, as revised in 2000 and 2008. The authors state that they have obtained appropriate institutional review board approval or have followed the principles outlined in the Declaration of Helsinki for all human or animal experimental investigations. In addition, for investigations involving human subjects, informed consent has been obtained from the participants involved.
Data security and privacy AI in Healthcare代写
Berg Interrogative Biology delivers solutions ranging from discovery-led in-house R&D programs, drug development through clinical and non-clinical research and integrated research services to leading global pharmaceutical and biotechnology companies. Their quest for innovation required them to prevent their confidential intellectual property, research and legal agreements from unauthorized access.
Usability and Acceptance
The study provides data in support of FLNB and LY9 as biomarkers with potential to improve screening outcomes when used in combination with PSA. Efforts are currently underway in large patient cohorts to validate the clinical utility of FLNB and LY9 as candidate biomarkers for use in the screening of prostate cancer with correlate to the Gleason score to enable more instructive data to the practitioner.
Outcome and limitations
The report provided evidence to support the use of a novel data-driven strategy to identify causal markers of PrCa. Using a combined in vitro and in silico approach, FLNB and KRT19 were identified as potential biomarkers for PrCa and FLNA was found to be causally linked to FLNB. These data suggest that the combined use of both markers in a panel may be necessary for detecting PrCa. The combined use of both markers in a panel in conjunction with prostate-specific antigen may potentially yield better predictive power for detection of prostate cancer than prostate-specific antigen alone.
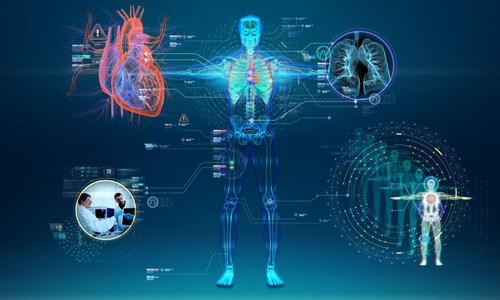
Case Study #4 Gene Expression Assay and Watson for Oncology for Optimization of Treatment in ER-positive AI in Healthcare代写
All patients underwent breast conserving surgery or total mastectomy and also a lymph node biopsy. Formalin-fixed paraffin-embedded (FFPE) tissue samples were derived from patients with primary ER-positive and HER2-negative breast cancer. All FFPE tumor blocks were collected at the time of surgery prior to therapy. Total RNA was extracted from FFPE tissue sections using a silica-based, fully automated isolation method. All samples were subjected to quantitative one-step SuperScript III PLATINUM reverse transcriptase PCR with ROX.
Quality and Safety
IBM Watson for Oncology is comprised of three integrated components, including: safety document management. Training and skills management and safety process improvement analysis. These components will help deliver safety decisions to companies through an improved method of collecting. Assessing and monitoring from various big data sources, including anonymised extensive electronic databases.
Data Security and Privacy AI in Healthcare代写
IBM Watson utilizes IBM Cloud Security with complete pervasive encryption, AI + automation and integration to stay ahead of threats. This cloud system provides prompt insight to help fight through thousands or more daily alerts to drastically reduce response times.
Usability and Acceptance
WFO results were assessed both with and without EPlin. With the WFO alone assessments, chemotherapy was recommended rather than endocrine therapy. Based on results with the WFO-EPlin combination, recommendations for chemotherapy were reduced from 78 to 23 patients. Chemotherapy was added by WFO in cases with high EPclin scores. This cognitive computing system help categorize patients suitable for either endocrine therapy alone or with added chemotherapy. This system has been excepted in this case study because it gave much promise to oncologists in making intensive and evidence based treatment decisions.
Outcome and Limitations AI in Healthcare代写
Watson for Oncology without gene expression assay was unable to determine the groups of patients that did not require chemotherapy. Real clinical practice and WFO without GEA sustained a report of 23.2% of the patient group. On the other hand, the results of WFO with GEA showed good applicability. Sensitivity, specificity, positive predictive and negative predictive values of WFO with GEA were 100%, 80%, 61% and 100%,respectively. However, the use of different genomic assessment tools could lead to calculations errors in the IBM formula. Keep in mind that at the end of it all, clinicians are ultimately responsible for their treatment. AI may deliver genomic information but it requires further development when applying effective implementation.
更多其他:研究论文代写 Academic代写 Review代写 网课代修 代写CS 数据分析代写 润色修改 代写案例 Case study代写 心理学论文代写 毕业论文代写 论文代写 Essay代写
您必须登录才能发表评论。