多元回归分析代写 Lecture 1: Multivariate Regression Analysis
多元回归分析代写 Under these assumptions we have the ordinary least square (OLS) estimator:The matrix
![]()
is often referred to as the projection matrix…
Unknown parameters:
Assumptions:
(Note
is constant)
Under these assumptions we have the ordinary least square (OLS) estimator:
The matrix
is often referred to as the projection matrix
Sampling properties of the OLS estimator:
- we look at the property of unbiasedness for
- The variance-covariance matrix for
is given by
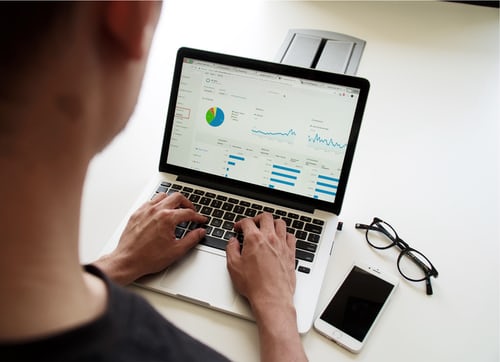
Given the unbiased property, the next question concerns comparison with other linear unbiased estimator. The Gauss-Markov theorem states:
Thus, BLUE
Having obtained a point estimator for the unknown parameter , we now turn to estimating
Where T is the number of observations and K is the number of explanatory variables.
is an unbiased estimator for
t-value=
Summary: 多元回归分析代写
- First, calculate
- Second, using the estimated
, calculate
- Third, generate t values for each parameter
- Finally, analysis your results.