Question 1
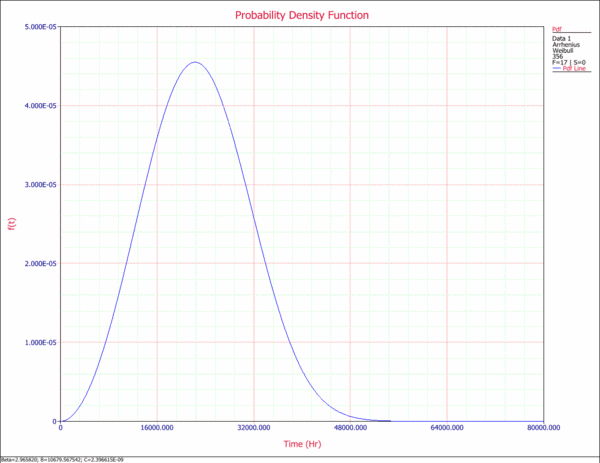
Normal Probability Density Function代写 (a) the Normal Probability Density Function with .Let , then plot with x and y, the result is shown in Fig1-1.
(a) the Normal Probability Density Function with .
Let , then plot with x and y, the result is shown in Fig1-1. This function is also called standard normal distribution.
Fig1-1 pdf of normal distribution with
(b) graph a normal distribution with , where the result is shown in Fig1-2.
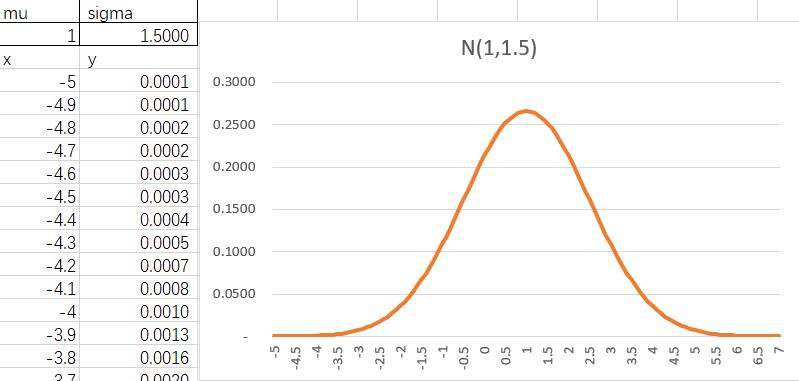
Fig1-2 pdf of normal distribution with
(c) Let , x is same as in part(a). Normal Probability Density Function代写
then plot t and y ()
the result is shown in Fig1-3.
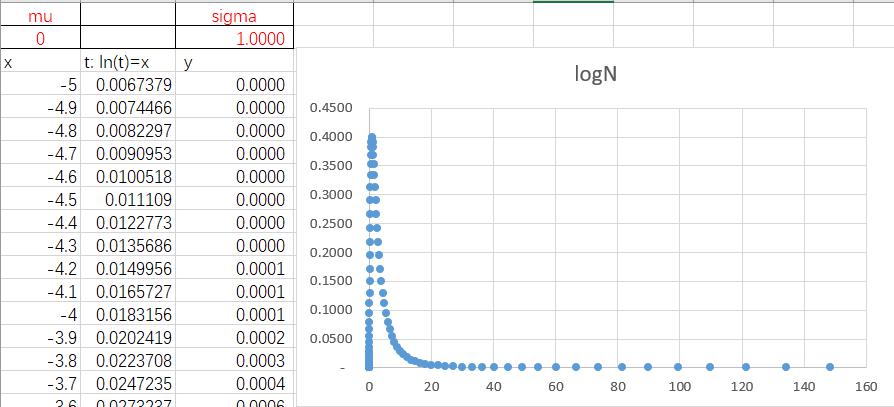
Fig1-3 pdf of log-normal
(d) plot another log-normal distribution. the result is shown in Fig1-4.
Fig1-4 pdf of log-normal .5
Question 2 Normal Probability Density Function代写
(a)
step 1: select the SP200 Index price and Yields on Australian 10-year government bond from 2017-07-06 to 2018-07-06. Data volume of yield on government bond are less than that of SP200 Index. Then, alignment time axis based on the time axis of the bond at first.
Step 2: graph the levels of the above data.
Fig2-1 levels
Step 3: graph the continuous return of SP200 index, . the yield curve of bond is the return curve, which has given in Fig2-1, so, in this step only give the return of SP200 index. The result is shown in Fig2-2.
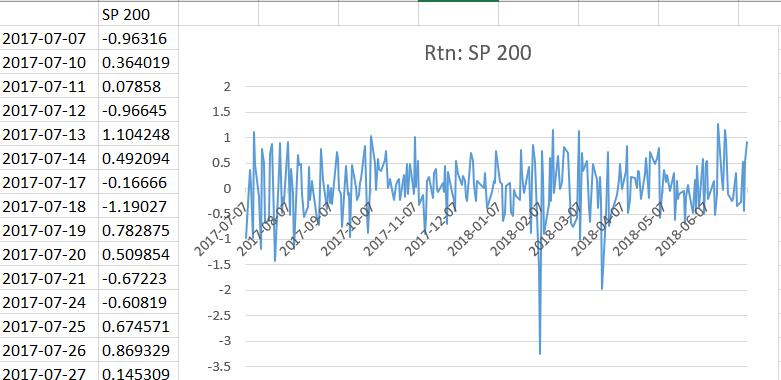
Fig2-2 return
Step 4: scatter plot is shown in Fig2-3.
Fig2-3 scatter plot
Step 4: time series plot is shown in Fig2-4.
Fig2-4 time-series plot
Step 5: it looks like that SP200 and Government bond are independent. As shown in Fig2-4, when SP200 went up, sometimes yield on Government bond went down (2017-10-03~2017-11-06), and sometimes yield on Government bond went up (2018-04-03~2018-04-24). Normal Probability Density Function代写
(b) using function in Excel, we can calculate the correlation coefficient for levels is 0.0652, and the correlation coefficient for returns is -0.0601.
The result shows that the correlation coefficients between MKT and RATE are nearly zero. During a relative long time more than one month, when the MKT reaches a local peak, the RATE may go up. But it still happened both stock market and bond market are bullish.

(c) use function in Excel to count the corresponding numbers.
MKT
RATE |
|||||
Rise | No change | Fall | Total | ||
Rise | 79 | 0 | 52 | 131 | |
No change | 1 | 0 | 1 | 2 | |
Fall | 56 | 0 | 64 | 120 | |
Total | 136 | 0 | 117 | 253 |
(d) Calculate the joint probability distribution and the marginal probability distributions for the data.
Joint probability distribution: Normal Probability Density Function代写
P {MKT=’Rise’, RATE=’Rise’} = 79/253;
P {MKT=’Rise’, RATE=’No change’} = 1/253;
P {MKT=’Rise’, RATE=’Fall’} = 56/253;
P {MKT=’No change’, RATE=’Rise’} = 0/253=0;
P {MKT=’No change’, RATE=’No change’} = 0/253=0;
P {MKT=’No change’, RATE=’Fall’} = 0/253=0;
P {MKT=’Fall’, RATE=’Rise’} = 52/253;
P {MKT=’Fall’, RATE=’No change’} = 1/253;
P {MKT=’Fall’, RATE=’Fall’} = 64/253;
Marginal probability distribution:
P {MKT=’Rise’} = 136/253;
P {MKT=’No change’} = 0/253=0;
P {MKT=’Fall’} = 117/253;
P {RATE=’Rise’} = 131/253;
P {RATE=’No change’} = 2/253;
P {RATE=’Fall’} = 120/253;
(e) as P {MKT=’Rise’, RATE=’Rise’} + P {MKT=’No change’, RATE=’No change’}+ P {MKT=’Fall’, RATE=’Fall’} =143/253 > 1/2.
RATE and MKT are weekly positive dependent with each other.
(f) conditional probability distribution:
P {MKT=’Rise’| RATE=’Rise’}
= P {MKT=’Rise’, RATE=’Rise’}/ P {RATE=’Rise’} =79/131;
P {MKT=’No change’| RATE=’Rise’}
= P {MKT=’No change’, RATE=’Rise’}/ P {RATE=’Rise’} =0;
P {MKT=’Fall’| RATE=’Rise’}
= P {MKT=’Fall’, RATE=’Rise’}/ P {RATE=’Rise’} =52/131.
Question 3 Normal Probability Density Function代写
(a) the standard deviation of daily return of SP200 is 0.0055.
The 95 % confidence interval for the daily returns for MKT is

(b) hypothesis test:
testing statistic:
Level of Significance: a = 0.05
Decision rule:
Computation:
Conclusion: so, we would not reject at significance level .
they would not invest further in Australian share market using this rule.
(c) hypothesis test:

testing statistic:
Level of Significance: a = 0.05
Decision rule:
Computation: JB=181.63 > 5.99
Conclusion: so, we would reject at significance level .
As in part(a), we suppose that the daily return is normally distributed, so the result is not accurate, but with large number theory, we also can conclude that the result shown in part(a) is approximately true.
As in part (b), we take student t-distribution as the testing statistic’s approximated distribution, so there is no problem with the result.
(d) runs test
Hypothesis test: 2-tail test
testing statistic:
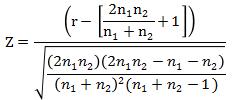
Level of Significance: a = 0.05
Decision rule:
Conclusion: so, we accept at significance level . That rises and falls are independent.
Question 4
(a) at first calculate the simple daily return of SP200 index , and the corresponding daily return of bond .
use Excel add-ins data analysis- regression to conduct this process.
SUMMARY OUTPUT | ||||||
regression summary | ||||||
Multiple R | 0.02725 | |||||
R Square | 0.00074 | |||||
Adjusted R Square | -0.00324 | |||||
stdev | 0.00553 | |||||
number of observations | 253 | |||||
ANOVA | ||||||
df | SS | MS | F | Significance F | ||
regression | 1 | 0.0000 | 0.0000 | 0.1865 | 0.6662 | |
residual | 251 | 0.0077 | 0.0000 | |||
Total | 252 | 0.0077 | ||||
Coefficients | stdev | t Stat | P-value | Lower 95% | Upper 95% | |
Intercept | 0.0003 | 0.0003 | 0.8811 | 0.3791 | -0.0004 | 0.025725 |
X Variable 1 | -0.0672 | 0.1556 | -0.4318 | 0.6662 | -0.3737 | 6.235623 |
Also, using Excel function =INTERCEPT(known y’s ,known x’s)
and =SLOPE(known y’s ,known x’s), we can get
Which is same as using regression method.
As shown in regression summary, ei2 = 0.007868.
The regression equation is MKTt = 0.0003–0.0672* RATEt Normal Probability Density Function代写
(b) use the continuous return of SP200 index, then re-estimate the regression equation.
SUMMARY OUTPUT | ||||||
regression analysis | ||||||
Multiple R | 0.0278 | |||||
R Square | 0.0008 | |||||
Adjusted R Square | -0.0032 | |||||
std | 0.0055 | |||||
observations | 253 | |||||
ANOVA | ||||||
df | SS | MS | F | Significance F | ||
regression | 1 | 0.0000 | 0.0000 | 0.1942 | 0.6598 | |
residuals | 251 | 0.0077 | 0.0000 | |||
total | 252 | 0.0077 | ||||
Coefficients | std | t Stat | P-value | Lower 95% | Upper 95% | |
Intercept | 0.0003 | 0.0003 | 0.8350 | 0.4045 | -0.0004 | 0.0010 |
X Variable 1 | -0.0688 | 0.1560 | -0.4407 | 0.6598 | -0.3761 | 0.2385 |
The equation is MKTt = 0.0003–0.0688* RATEt
(c) use excel Function , for both continues MKT return and RATE.
We can get
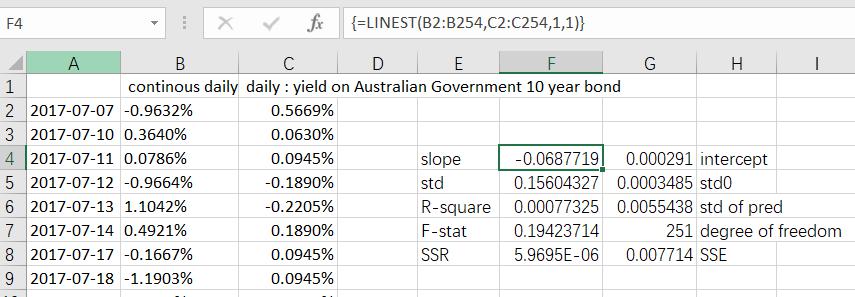
(d) in part (a) using simple return of Mkt data, in part(b) and part(c) using continuous return of MKT data, there are small difference in coefficients of the equation. But all displays that there are weak negative relationship between return of MKT and return of RATE.
Question 5
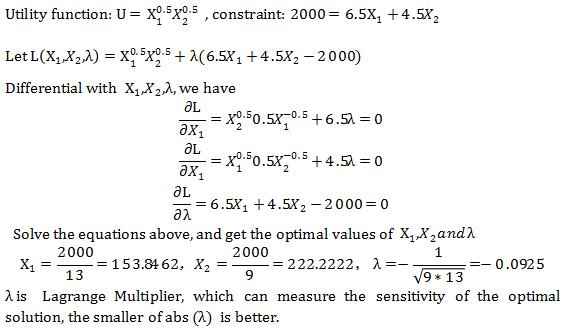
Using Excel solve add-ins we can get the result as below, which is consistent with the result using Lagrange Multiplier Method.
Question 6
(a) dimensions of A, B and C
(e) using function in Excel to compute the matrix product.
(f) verify that :
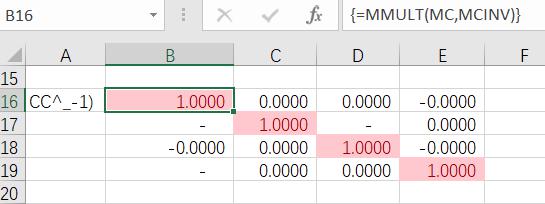
Question 7
Step1: calculate the continuous return of each security. Because code: 9305KL and 9217CU has missing values, so take out those two securities. As 2017-12-25, 2017-12-26, 2018-1-1, 2018-1-26, 2018-3-30, 2018-4-2, 2018-4-25,2018-6-11 are Non-trading days, take out the data of those days. then we have 253 days data with 198 tickers.
Only those 7 stocks are classified in Oil& Gas.
According to the instructions, we can use Excel Solver Tool to find the minimum risk for portfolios for various expected return (about 10 risk-return data)
By changing the values in Twts, we minimum Prsk with constraints :
Wtcn=1, Twts>=0, ret=predefined return of the portfolio.
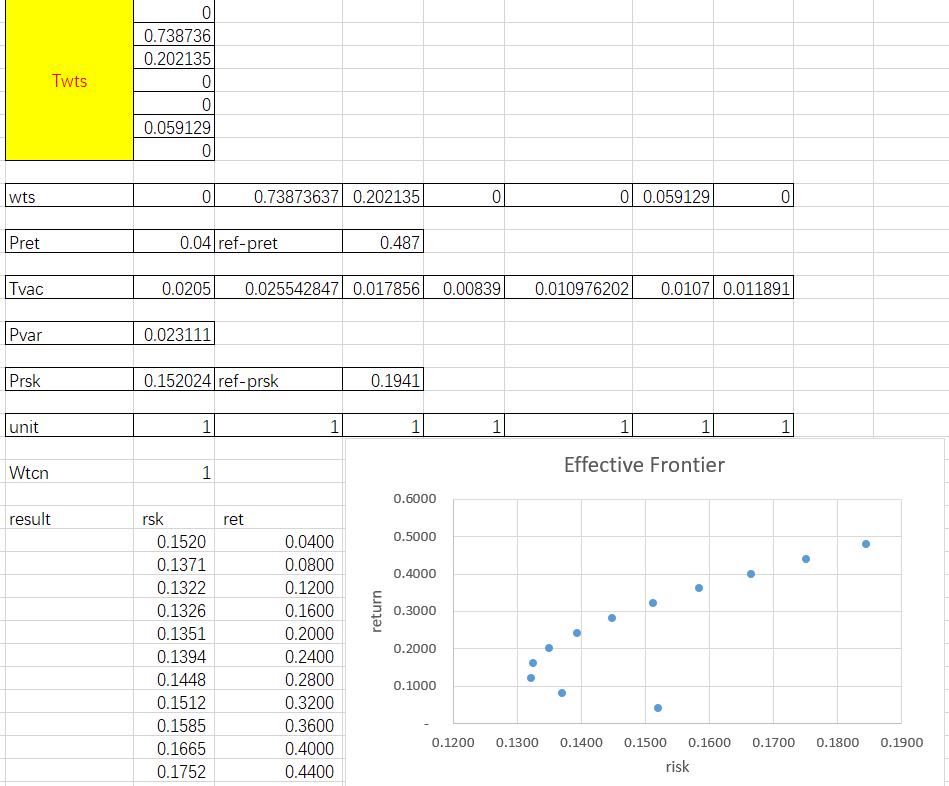
The result tells that portfolio’s risk is not linear with expected return. When we try expected returns smaller than 0.12, the relative portfolio risk will be increase as the decrease of expected returns; and when we try expected returns larger than 0.12, the relative portfolio risk will increase as the increase of expected returns.
So we should choose the wts that have larger return given the same risk.
Question 8 Normal Probability Density Function代写
Step 1: select data of the financial sector components in sheet: Raw Prices for constituents.
And calculate the continuous return, take out non-trading days, and take out the security (9305KL) that has Nas in return.
CODE | BETA | ER | BETASQ | VAR |
950706 | 1.1884 | -0.0000 | 1.4122 | 0.0065 |
905209 | 1.1918 | -0.0001 | 1.4203 | 0.0066 |
981800 | 1.2052 | -0.0005 | 1.4524 | 0.0098 |
316588 | 1.3554 | -0.0000 | 1.8371 | 0.0102 |
675493 | 0.6445 | -0.0001 | 0.4154 | 0.0135 |
675667 | 0.6164 | 0.0004 | 0.3800 | 0.0071 |
675705 | 0.9260 | 0.0007 | 0.8575 | 0.0066 |
691601 | 0.7410 | 0.0003 | 0.5491 | 0.0105 |
691992 | 0.9447 | 0.0013 | 0.8924 | 0.0116 |
280141 | 0.5901 | 0.0006 | 0.3482 | 0.0104 |
314054 | 1.0925 | -0.0004 | 1.1937 | 0.0074 |
502144 | 0.4248 | 0.0007 | 0.1805 | 0.0081 |
887937 | 0.4720 | 0.0001 | 0.2228 | 0.0079 |
297124 | 1.0108 | 0.0007 | 1.0218 | 0.0096 |
263898 | 1.2014 | 0.0000 | 1.4434 | 0.0148 |
916763 | 0.7497 | 0.0003 | 0.5620 | 0.0087 |
901843 | 0.9599 | 0.0007 | 0.9213 | 0.0122 |
26502C | 0.7629 | 0.0009 | 0.5820 | 0.0100 |
503798 | 0.7578 | 0.0000 | 0.5743 | 0.0088 |
901842 | 1.0184 | -0.0003 | 1.0372 | 0.0064 |
998066 | 0.9366 | -0.0009 | 0.8773 | 0.0126 |
502192 | 0.9236 | 0.0009 | 0.8531 | 0.0112 |
675492 | 0.7795 | -0.0014 | 0.6077 | 0.0115 |
27952T | 1.0277 | -0.0002 | 1.0561 | 0.0129 |
930388 | 0.9346 | -0.0008 | 0.8734 | 0.0108 |
879286 | 1.2004 | -0.0004 | 1.4408 | 0.0135 |
905506 | 0.7102 | -0.0003 | 0.5044 | 0.0092 |
28516K | 1.2524 | 0.0005 | 1.5684 | 0.0120 |
28836N | 1.4916 | -0.0007 | 2.2250 | 0.0137 |
754824 | 0.8594 | 0.0002 | 0.7386 | 0.0093 |
503969 | 0.7122 | 0.0008 | 0.5072 | 0.0086 |
36003D | 0.9746 | 0.0008 | 0.9498 | 0.0092 |
50579C | 1.3517 | 0.0006 | 1.8270 | 0.0209 |
507749 | 0.5084 | 0.0006 | 0.2585 | 0.0071 |
865438 | 1.2219 | 0.0013 | 1.4931 | 0.0087 |
87867P | 0.4081 | 0.0006 | 0.1666 | 0.0078 |
89592Q | 0.9774 | 0.0002 | 0.9553 | 0.0135 |
8851HK | 1.0237 | 0.0010 | 1.0479 | 0.0147 |
2640K4 | 0.2664 | 0.0003 | 0.0709 | 0.0083 |
9105LQ | 1.0780 | 0.0045 | 1.1622 | 0.0323 |
51280P | 1.4084 | -0.0003 | 1.9835 | 0.0146 |
362569 | 0.8562 | -0.0005 | 0.7330 | 0.0132 |
93650L | 0.3855 | 0.0005 | 0.1486 | 0.0096 |
8866ZY | 0.7178 | 0.0004 | 0.5152 | 0.0092 |
8898YZ | 1.0142 | -0.0007 | 1.0286 | 0.0169 |
8875U5 | 0.6669 | 0.0002 | 0.4447 | 0.0108 |
9387VV | 1.2033 | -0.0005 | 1.4480 | 0.0130 |
779763 | 0.5520 | 0.0001 | 0.3047 | 0.0095 |
95378H | 0.5718 | 0.0007 | 0.3269 | 0.0157 |
27917F | 1.2192 | -0.0000 | 1.4863 | 0.0115 |
7749ZN | 0.4338 | -0.0001 | 0.1882 | 0.0074 |
Conduct function in Excel to estimate the SML, and we find the slope is -0.0686, and the intercept is 0.1235.
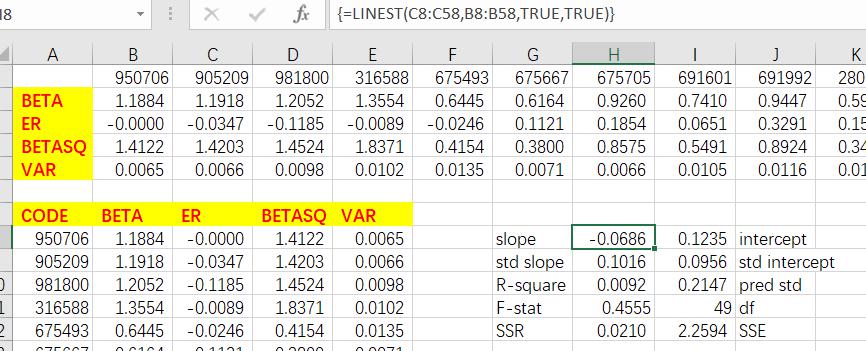
Also, we conduct linear regression using data analysis add-in in excel. Normal Probability Density Function代写
SUMMARY OUTPUT | ||||||
regression analysis | ||||||
Multiple R | 0.0959703 | |||||
R Square | 0.009210299 | |||||
Adjusted R Square | -0.011009899 | |||||
pred std | 0.214734946 | |||||
observations | 51 | |||||
ANOVA | ||||||
df | SS | MS | F | Significance F | ||
regression | 1 | 0.021004 | 0.021004 | 0.4555 | 0.502907 | |
residuals | 49 | 2.259444 | 0.046111 | |||
Total | 50 | 2.280447 | ||||
Coefficients | std | t Stat | P-value | Lower 95% | Upper 95% | |
Intercept | 0.123518199 | 0.095569 | 1.292446 | 0.202264 | -0.06854 | 0.315572 |
BETA | -0.068593656 | 0.101634 | -0.67491 | 0.502907 | -0.27284 | 0.135648 |
Then, conduct regression process, and we get the following result.
SUMMARY OUTPUT | ||||||
regression analysis | ||||||
Multiple R | 0.5189451 | |||||
R Square | 0.26930402 | |||||
Adjusted R Square | 0.22266385 | |||||
pred std | 0.18829095 | |||||
observations | 51 | |||||
ANOVA | ||||||
df | SS | MS | F | Significance F | ||
regression | 3 | 0.614134 | 0.204711 | 5.774079 | 0.001906 | |
residuals | 47 | 1.666314 | 0.035453 | |||
Total | 50 | 2.280447 | ||||
Coefficients | std | t Stat | P-value | Lower 95% | Upper 95% | |
Intercept | -0.1589226 | 0.210921 | -0.75347 | 0.454927 | -0.58324 | 0.265396 |
BETASQ | -0.1511275 | 0.277209 | -0.54517 | 0.588211 | -0.7088 | 0.406546 |
VAR | 26.9600738 | 6.666663 | 4.044013 | 0.000194 | 13.54848 | 40.37167 |
BETA | 0.06238299 | 0.49629 | 0.125699 | 0.900507 | -0.93602 | 1.060789 |
The coefficient of VAR’s p-value is less than 0.05, which means significant. But the coefficient for BETA and BETASQ is not significant. With more explanatory variables like BETASQ and VAR, the adjusted coefficient of determination (namely adjusted R-square have improved a lot, the latter regression’s adjusted R-square is 0.22>-0.01.
t-statistic for BETA is 0.125, for BETASQ is -0.54 and for intercept is -0.75, so the absolute values of these three variables’ t-statistic is less than the critical value t(47, 0.95) =1.68, so BETA, BETASQ, intercept don’t have significant impact on expected returns. As t-statistic for VAR is 4.04>1.68, so, VAR has a significant impact on expected returns.
regression function’s coefficients of SML are both insignificant, we doubt that daily returns may have too much noise, maybe monthly returns will perform better. The result in SML regression function shows that the intercept>0,which means the risk-free rate is nearly 0.12, and BETA’s coefficient=-0.06<0, which means the risk-premium of MKT is less than 0.
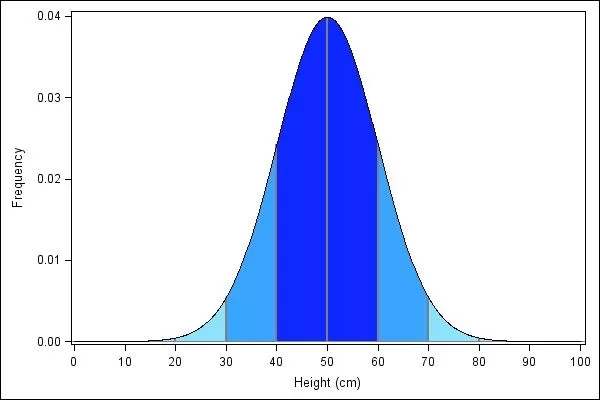
更多其他:prensentation代写 文学论文代写 商科论文代写 Resume代写 文学论文代写 商科论文代写 艺术论文代写 人文代写
您必须登录才能发表评论。