Artificial Intelligence and Future of Human Workforce
Name
Institution
Artificial Intelligence and Future of Human Workforce
AI Technology代写 Artificial Intelligence and Future of Human Workforce.Background of AI and it relationship to organizational decision-making.
Introduction
The modern AI development has surpassed the human cognitive abilities and exert pressure in people of potential dehumanization of the future workplace. AI technologies have become visible and in a rapid momentum by the recent developments such as Watson in IBM and AlphaGo in Google. Although there are variations to the concept of AI, generally, it means systems that are intelligent and can learn (Russel, Norvig, & Intelligence, 1995). It constitutes a set of tools, techniques, and algorithms that work together. AI Technology代写**范文
AI categories have common elements that build up their cognitive abilities, including machine vision, machine learning, and natural language processing. As such, looking at these features that are inherent to humans and that has continued to augment human work means that AI will continue to automate repetitive and physical tasks as well as quantitative functions such as programming and data science. AI Technology代写**范文
However, this will only try to push the human skillset ladder to exhibit skills such as creativity, social abilities, empathy, and sense-making, which fundamentally lacks in AI. Therefore, AI is making the workplace more human through human-machine symbiotic in organizational decision-making.
Background of AI and it relationship to organizational decision-making AI Technology代写
Evaluate
In this context, an excellent example of AI IBM’s Watson can be evaluated on its essential elements (Captain, 2017). The system has natural language processing to understand human language and has various interpretations. Watson has machine learning capability to learn and make intelligent solutions through interaction and experience acquired from the pool of data acquired over time. AI Technology代写**范文
It has used machine learning techniques coupled with access to vast records of medical data to learn and diagnose cancer. Medical staff uses Watson as a compliment that is halfway towards cancer treatment. Finally, Watson uses machine vision to process MRI images and mark different small parts of the brain for use by the doctors.
Higher-learning capabilities AI Technology代写
The modern AI systems like Watson have higher-learning capabilities derived from knowledge-based tasks. These domains were previous exclusive abilities to humans. Wladawsky-Berger (2017) noted that knowledge-based tasks performed by white-collar workers were considered immune from automation. The reason why some of these tasks are automated is that IT technologies are advancing to the extent of functioning as semi-autonomous decision-makers (Davenport & Kirby, 2016). Courtesy of smart technologies, the postindustrial economies are coming to the age of second machine age that is replacing human beings in various fields.
Proliferation of AI systems AI Technology代写
Therefore, the proliferation of AI systems has posed vexing questions to organizations about its future implication to the workforce. MacCrory, Westerman, Alhammadi, and Brynjolfsson (2014) noted that “for any given skill one can think of, some computer scientist may already be trying to develop an algorithm to do it.” Tech tycoons like Elon Musk envision a situation whereby AI will have significant disruptions to the human workforce (Kalev, 2016). AI Technology代写**范文
AI systems are considered to be the epicenter of future work automation. Notably, experts and organizational managers are perceiving it as the driver to the transformation of decision-making. New Vantage Partners (2017) suggested that AI is continuing to disrupt the business landscape, and therefore, managers have to plan on future investment in AI-related technologies.
Fascination with AI technologies AI Technology代写
However, there is a need to put the fascination with AI technologies and their likelihood to take over the human workforce into context.In 1930, John Maynard Keynes, through his article “Economic Possibilities for our Grandchildren,” envisioned technology as the disease terming it “technology disease” (Wladawsky-Berger, 2017). Recently, Zuboff (1988) presented IT as the smart technology of the future. AI Technology代写**范文
Zuboff noted that IT promises a workplace that is transparent and more rewarding, but if used exclusively, risk human control. As such, work automation has been a subject of concern on its impact on human work.
Views AI Technology代写
The hyperbole surrounding AI technologies that have caused a perception that they will outdo human beings at work in the future is also an overhyped proposition. The inflated views on AI are not new as Simon (1965), a cognitive scientist, predicted that machines might take over all human work by 1985. Marvin Minsky also made an audacious projection that in less than eight years from 1970. AI Technology代写**范文
There will be an AI system with intelligence closer to average human beings and that it will learn in few months, and over the years, its intelligence will be incalculable (King & Grudin, 2016). However, the past and current discourse on AI’s impact on the human workplace focus on unique abilities that human beings have that machines systems are acquiring and hence fail to focus on the synergy that is created if humans and AI work together symbiotically.
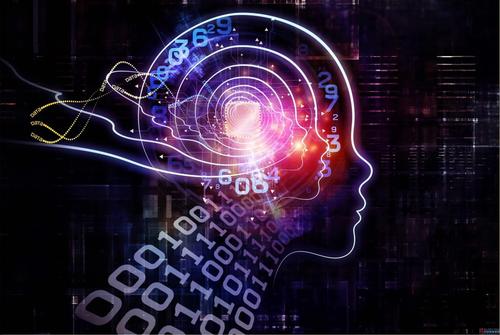
Human-machine complementarity AI Technology代写
Complementarity
As such, the argument in this article vested on the statement that computers working together with a human can do better than either alone (Campbell, 2016). Therefore, a critical approach to complementarity in humans and AI in an organization’s decision-making is essential. A good scenario is that of a game of chess where the abilities of chess masters to predict and process contingencies are limited to their cognitive abilities; they only consider 100 contingencies, which only make up10 percent of the possible moves (Simon, 1982). AI Technology代写**范文
AI does not have cognitive bound and hence was able to defeat the grand Gary Kasparov. Although his defeat predicted the end of a chess game, Kasparov came up with a new version of chess league where the chess master was neither AI nor human. He termed them centaurs that constituted a partnership of humans with AI. This example of chess envisions a complementary future of AI and human workforce; that is, AI and humans will offer different abilities but complementary to attain a particular organizational goal.
Symbiotic nature AI Technology代写
Additionally, the synergy created during a collaboration between AI and human cannot only be seen in the game of chess but also elsewhere. A study on cancer detection using images of lymph nodes cell found that exclusive use of AI caused a 7.5 percent error rate while that of pathologies gave an error rate of 3.5 percent. However, when the inputs from both were considered, the error rate reduced to 0.5 percent (Wang, Khosla, Gargeya, Irshad, & Beck, 2016). AI Technology代写**范文
The findings of this study confirmed the vision by J. C. R. Licklider that the future workforce will a symbiotic relationship between humans and AI, where strengths one complements the weaknesses of the other. The symbiotic nature of AI and humans is on the horizon. Nonetheless, the concern is how the two can be used complementarily in organizational decision-making.
Approaches AI Technology代写
The question on the complementarity of human and AI can be addressed by establishing the differences between analytical and intuitive approaches to organizational decision-making as well as organizational challenges in making decisions, including uncertainty, complexity, and equivocality (Choo, 1991; Simon, 1982). Analytical and intuitive approaches were distinguished through analyzation of studies on the daily practices of managers and workers and how they process information and make decisions (Dane, Rockmann, & Pratt, 2012). AI Technology代写**范文
When humans use analytical skills, they engage in methodological, laborious data collection, and analysis. Individuals engage in conscious reasoning and logical deliberation in the analytical approach. The ability of AI on problem-solving can be used to support human analysis intend rather than applying intuitive decision-making.
Intuition AI Technology代写
On the other hand, human decision making does not mainly rely on data collection and analysis but instead on the use of intuition. Intuition is the use of the subconscious mind and knowledge to decide without having to make logical reasoning and inferences (Sadler-Smith & Shefy, 2004). It is more of a “gut feeling” about a decision. The use of intuition in making decisions involves imagination, creativity, sensitivity, rumination, and intelligence (Bishop, 2000). AI Technology代写**范文
Intuition enables humans to use known practices, experience, and judgment to come up with a solution without conscious attention. Thus, the analytical approach makes decisions based on the collection of information while the intuitive approach views the problem holistically and in the abstract. Both approaches are not mutually exclusive and hence used in parallel to make a more informed decision.
Common-sense AI Technology代写
Therefore, AI systems are suitable in the analytical approach in decision-making but are not capable of making common-sense on the decision like a human can (Guszcza, Lewis, & Evans-Greenwood, 2017). Also, AI is not reliable in situations of high uncertainty and unpredictability (Brynjolfsson & McAfee, 2012). Human is more reliable on tasks that require the use of common sense. That is, humans rely more on subjective judgment and hence suitable for jobs that require an intuitive approach. On the other hand, AI is more effective in an analytical approach to making decisions that require data analysis to make a decision.
Uncertainty AI Technology代写
Define
Choo (1991) defined uncertainty as a lack of enough information about the choices and results and hence makes interpretation and making a decision of a particular phenomenon difficult. Uncertainty occurs when the management lacks adequate information about the organizational external and internal environment. AI uses probabilistic and statistical inferences to find the relationships between various variables. Human workers use AI findings and predictions to make informed decisions. AI Technology代写**范文
Predictive analytics are mainly used to provide new insight into customers or operations. As such, big corporations such as Deloitte and McKinsey use AI systems to monitor organizational environments and hence act as semi-autonomous strategy articulation. Managers can use the AI system to make detection of issues early before they negatively impact the organization.
Ambiguity AI Technology代写
On the other hand, there are times when the organization is faced with an unprecedented situation that ends up creating overwhelming ambiguity. In such circumstances where no data is available, an intuitive approach to decision-making is preferable. Such issues are global crisis or minor technical hitches can be unprecedented that the results are not predictable based on the information-centric rational processes. AI systems cannot be used to tackle novel problems such as the current COVID-19 global health crisis (Guszcza, Lewis, & Evans-Greenwood, 2017; Unicef, 2020). AI Technology代写**范文
As such, human forms the basic part of problem-solving using intuition that is rooted in life experience. Intuitive decision-making is prioritized over an analytical approach, especially when a major decision is to be made by seniors. It allows for creative decision-making in an organization which is inapplicable when it comes to AI systems because they lack inner logic and subconscious patterns (Gardner & Martinko, 1996). Thus, there is no likelihood of AI technologies mimicking human decision-making abilities in areas that require intuition.
Complexity AI Technology代写
A complex task is one that has many interrelated and disjointed variables or elements. Such tasks require high processing power that is beyond the cognitive speed of human beings. Modern AI machines have capabilities to do the complex quantitative, computation, and analytical tasks. AI has reduced complex tasks by establishing the existence of causality and the cause of action using various possibilities (Marwala, 2015). AI systems are complemented by big data analytics to come up with better approaches that give human decision-makers comprehension of data analysis. AI Technology代写**范文
The contribution of AI can be observed in a wide range of business applications, from credit analysis, social media analytics, digital marketing, to real estate. The emergence of deep learning has enabled machines to learn from raw data that is large and complex. The ability to find interrelatedness in large raw data is beyond human ability. The synergy is hence created when the speed of the AI system collecting and analyzing data is combined with human intuitive and creative abilities.
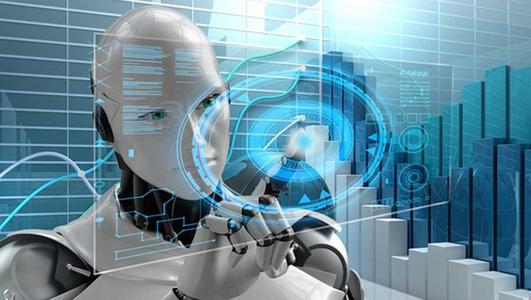
Equivocality AI Technology代写
Opinions
According to Weick and Roberts (1993), equivocality is the existence of two but divergent opinions regarding a particular problem. It occurs from differences in interests among the customers, shareholders, and policymakers. As such, the organizational decision-making process shifts from being an impartial and objective process to being subjective and politically intended to satisfy various interest parties. AI Technology代写**范文
Rational decisions can be stymied by powerful interest groups affected by it. AI systems can be used to overcome the impact of different problem solutions. For instance, it can use social media sentiments regarding a specific issue both in the organization and outside and provide possible reactions from different parties affected by the decision.
Reason AI Technology代写
Nonetheless, equivocal decisions are predominantly made by human beings. The reason being, humans have superior abilities to decipher political, social, economic landscapes in and out of the organization and create a foundation for negotiations and implementation of the solutions. Although AI systems can develop a probable decision, they cannot make divergent opinions agree. Campbell (2016) noted that chess computers could make absurd and objective moves as long as it thinks it is the best move. Computers do not try to make sense when making decisions.
As such, the objective, impersonal approach in decision-making that machine use is not suitable in subjective, emotionally-charged, and contextually sensitive sentiments that exist in any organization.
Leader AI Technology代写
Additionally, every organization wants a leader that can come up with viable and objective decision and ability to communicate and persuade others. As such, a manager needs to be emotionally and socially intelligent, coupled with interpersonal skills that are essential practice. Also, informal leaders have a crucial role in making equivocal decisions. Cross, Borgatti, and Parker (2002) noted that informal leaders hold a better position to create consensus between conflicting interest groups because they have more knowledge, social ties, and skills in the organization. AI Technology代写**范文
An AI system cannot dissect the complex social systems that exist in organizations. Also, it impractical for a worker to follow the AI system the same way they can follow a rational manager. Therefore, a human will continue to have a comparative advantage over AI systems in the comprehension of convoluted social and political dynamics in an organization.
Not mutually exclusive AI Technology代写
Notably, the organizational workforce is characterized by uncertainty, complexity, and equivocality that are not mutually exclusive. Optimal decision-making in an organization is a balance between analytical and intuitive approaches. All decisions are a combination of both deduction and intuition, and therefore reliance on either one of them alone is not adequate. Overall, the symbiotic connection between humans and AI can be spelled out in two ways. AI Technology代写**范文
First, both can collaborate to solve different aspects of making a decision. In which, AI system solve complex interrelationship between variables and elements using analytical approach while human tackle those that involve uncertainty and equivocality using creativity and intuition. Secondly, a complex task that needs AI tackling can have uncertainty and equivocality. Thus, human has a role in almost all complex task handled by AI systems.
Conclusion AI Technology代写
AI advancement is a trigger for more human-machine complementarity. Technology comes with a new era of the division of labor and specialization. The relationship between the two can be perceived as that AI will take up the mundane and repetitive roles to allow for humans to be creative in dealing with other aspects of work. As such, this article goes beyond mere thinking that AI systems are here to take over the human workforce by countering it with the fact that AI system integration in organizations is purely based on comparative advantage. AI Technology代写**范文
AI systems are better in the analysis of complex tasks, while humans are better in tasks that require creativity and intuition. And since these elements of tasks are not mutually exclusive, human-machine symbiosis is not only necessary but also expedient. It will remain unquestionable of the human ability to make decisions that involve uncertainty and equivocality and that machines will always depend on humans for tasks that require subconscious decision heuristics to weigh options.
References AI Technology代写
B-G
Bishop, P. (2000). Synchronicity and intellectual intuition in Kant, Swedenborg, and Jung: The Edwin Mellen Press.
Brynjolfsson, E., & McAfee, A. (2014). The second machine age: Work, progress, and prosperity in a time of brilliant technologies: WW Norton & Company.
Cross, R., Borgatti, S. P., & Parker, A. (2002). Making invisible work visible: Using social network analysis to support strategic collaboration. California management review, 44(2), 25-46.
Choo, C. W. (1991). Towards an information model of organizations. The Canadian Journal of Information Science, 16(3), 32-62.
Captain, S. (2017). Can IBM’s Watson Do It All? Fast Company. Retrieved from https://www.fastcompany.com/3065339/can-ibms-watson-do-it-all
Campbell, M. (2016). 20 Years Later, Humans Still No Match for Computers on The Chessboard. NPR. Retrieved from http://www.npr.org/sections/alltechconsidered/2016/10/24/499162905/20-years-later-humans-still-no-match-for-computers-on-the-chessboard
Dane, E., Rockmann, K. W., & Pratt, M. G. (2012). When should I trust my gut? Linking domain expertise to intuitive decision-making effectiveness. Organizational Behavior and Human Decision Processes, 119(2), 187-194.
Davenport, T. H., & Kirby, J. (2016). Just How Smart Are Smart Machines? MIT Sloan Management Review, 57(3), 21.
Guszcza, J., Lewis, H., & Evans-Greenwood, P. (2017). Cognitive collaboration: Why humans and computers think better together. Deloitte University Press.
Gardner, W. L., & Martinko, M. J. (1996). Using the Myers-Briggs Type Indicator to study managers: A literature review and research agenda. Journal of Management, 22(1), 45-83.
K-S AI Technology代写
Kalev, L. (2016). Is Elon Musk Right and Will AI Replace Most Human Jobs? Forbes. Retrieved from https://www.forbes.com/sites/kalevleetaru/2016/11/08/is-elon-musk-right-and-will-ai-replace-most-human-jobs/#2d1c95bb60f4
King, J. L., & Grudin, J. (2016). Will Computers Put Us Out of Work? Computer, 49(5), 82-85.
Marwala, T. (2015). Causality, correlation, and artificial intelligence for rational decision making. Singapore: World Scientific.
MacCrory, F., Westerman, G., Alhammadi, Y., & Brynjolfsson, E. (2014). Racing with and against the machine: changes in occupational skill composition in an era of rapid technological advance. In Proceedings of the 35th International Conference on Information Systems., Auckland, NZ.
New Vantage Partners. (2017). Big Data Executive Survey 2017. Retrieved from http://newvantage.com/wp-content/uploads/2017/01/Big-Data-Executive-Survey-2017-Executive-Summary.pdf
Russell, S., Norvig, P., & Intelligence, A. (1995). Artificial Intelligence: A modern approach. Englewood Cliffs: Prentice-Hall.
Simon, H. A. (1982). Theories of bounded rationality. In H. A. Simon (Ed.), Models of bounded rationality. Behavioral economics and business organization (Vol. 1, pp. 408-423). Cambridge, MA: MIT Press.
Simon, H. A. (1965). The Shape of Automation for Men and Management. New York: Harper and Row.
Sadler-Smith, E., & Shefy, E. (2004). The intuitive executive: Understanding and applying ‘gut feel’ in decision-making. The Academy of Management Executive, 18(4), 76-91.
U-Z AI Technology代写
Unicef. (2020). Coronavirus disease (COVID-19). Retrieved from https://www.unicef.org/coronavirus/covid-19
Wang, D., Khosla, A., Gargeya, R., Irshad, H., & Beck, A. H. (2016). Deep learning for identifying metastatic breast cancer. arXiv preprint arXiv:1606.05718.
Weick, K. E., & Roberts, K. H. (1993). Collective mind in organizations: Heedful interrelating on flight decks. Administrative science quarterly, 357-381
Wladawsky-Berger, I. (2017). The Emerging, Unpredictable Age of AI. MIT Initiative on the Digital Economy. Retrieved from http://ide.mit.edu/news-blog/blog/emerging-unpredictable-age-ai
Zuboff, S. (1988). In the age of the smart machine: The future of work and power: Basic books.
更多其他:研究论文代写 数据分析代写 Case study代写 Report代写 Proposal代写 Capstone Projects Review代写 商科论文代写 文学论文代写 论文代写 Essay代写 Academic代写
您必须登录才能发表评论。